Stephen S. -T. Yau: Principles of Nonlinear Filtering Theory, Gebunden
Principles of Nonlinear Filtering Theory
(soweit verfügbar beim Lieferanten)
- Verlag:
- Springer Nature Switzerland, 12/2024
- Einband:
- Gebunden
- Sprache:
- Englisch
- ISBN-13:
- 9783031776830
- Artikelnummer:
- 12144905
- Umfang:
- 488 Seiten
- Gewicht:
- 891 g
- Maße:
- 241 x 160 mm
- Stärke:
- 32 mm
- Erscheinungstermin:
- 18.12.2024
- Hinweis
-
Achtung: Artikel ist nicht in deutscher Sprache!
Klappentext
This text presents a comprehensive and unified treatment of nonlinear filtering theory, with a strong emphasis on its mathematical underpinnings. It is tailored to meet the needs of a diverse readership, including mathematically inclined engineers and scientists at both graduate and post-graduate levels. What sets this book apart from other treatments of the topic is twofold. Firstly, it offers a complete treatment of filtering theory, providing readers with a thorough understanding of the subject. Secondly, it introduces updated methodologies and applications that are crucial in today's landscape. These include finite-dimensional filters, the Yau-Yau algorithm, direct methods, and the integration of deep learning with filtering problems. The book will be an invaluable resource for researchers and practitioners for years to come.
With a rich historical backdrop dating back to Gauss and Wiener, the exposition delves into the fundamental principles underpinning the estimation of stochastic processes amidst noisy observations-a critical tool in various applied domains such as aircraft navigation, solar mapping, and orbit determination, to name just a few. Substantive exercises and examples given in each chapter provide the reader with opportunities to appreciate applications and ample ways to test their understanding of the topics covered. An especially nice feature for those studying the subject independent of a traditional course setting is the inclusion of solutions to exercises at the end of the book.
The book is structured into three cohesive parts, each designed to build the reader's understanding of nonlinear filtering theory. In the first part, foundational concepts from probability theory, stochastic processes, stochastic differential equations, and optimization are introduced, providing readers with the necessary mathematical background. The second part delves into theoretical aspects of filtering theory, covering topics such as the stochastic partial differential equation governing the posterior density function of the state, and the estimation algebra theory of systems with finite-dimensional filters. Moving forward, the third part of the book explores numerical algorithms for solving filtering problems, including the Yau-Yau algorithm, direct methods, classical filtering algorithms like the particle filter, and the intersection of filtering theory with deep learning.
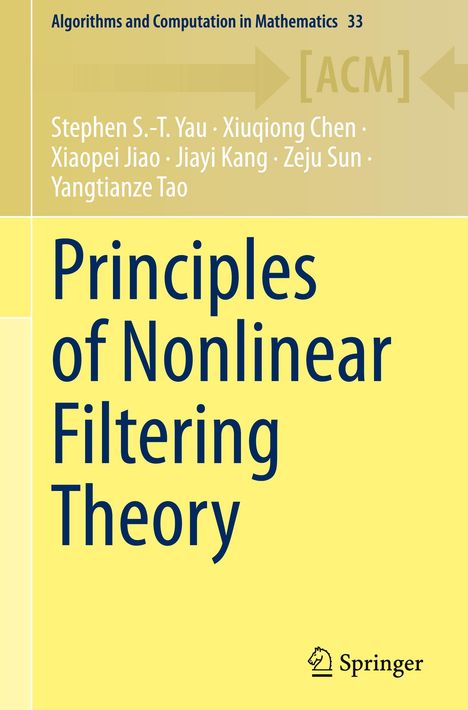