Anestis Antoniadis: Statistical Learning Tools for Electricity Load Forecasting, Gebunden
Statistical Learning Tools for Electricity Load Forecasting
(soweit verfügbar beim Lieferanten)
- Verlag:
- Springer International Publishing, 08/2024
- Einband:
- Gebunden, HC runder Rücken kaschiert
- Sprache:
- Englisch
- ISBN-13:
- 9783031603389
- Artikelnummer:
- 11944919
- Umfang:
- 244 Seiten
- Nummer der Auflage:
- 2024
- Ausgabe:
- 2024
- Gewicht:
- 535 g
- Maße:
- 241 x 160 mm
- Stärke:
- 19 mm
- Erscheinungstermin:
- 17.8.2024
- Hinweis
-
Achtung: Artikel ist nicht in deutscher Sprache!
Klappentext
This monograph explores a set of statistical and machine learning tools that can be effectively utilized for applied data analysis in the context of electricity load forecasting. Drawing on their substantial research and experience with forecasting electricity demand in industrial settings, the authors guide readers through several modern forecasting methods and tools from both industrial and applied perspectives ¿ generalized additive models (GAMs), probabilistic GAMs, functional time series and wavelets, random forests, aggregation of experts, and mixed effects models. A collection of case studies based on sizable high-resolution datasets, together with relevant R packages, then illustrate the implementation of these techniques. Five real datasets at three different levels of aggregation (nation-wide, region-wide, or individual) from four different countries (UK, France, Ireland, and the USA) are utilized to study five problems: short-term point-wise forecasting, selection of relevant variables for prediction, construction of prediction bands, peak demand prediction, and use of individual consumer data.
This text is intended for practitioners, researchers, and post-graduate students working on electricity load forecasting; it may also be of interest to applied academics or scientists wanting to learn about cutting-edge forecasting tools for application in other areas. Readers are assumed to be familiar with standard statistical concepts such as random variables, probability density functions, and expected values, and to possess some minimal modeling experience.
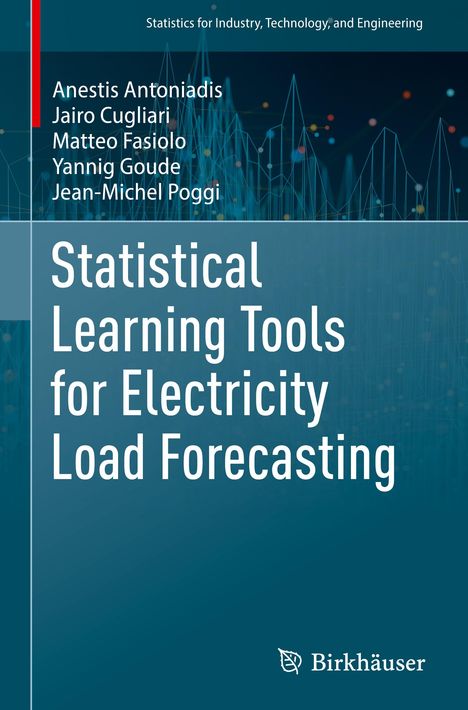